Solving Gen-AI's Last Mile Problem: “AI’s $600B question” - a Response
- Arindom Banerjee
- Aug 17, 2024
- 10 min read
Updated: Aug 22, 2024
Abstract:
The AI (Gen-AI) industry stands at a critical juncture, facing a staggering $600 billion gap between investments and realized value. This article challenges the status quo, arguing that the path to AI profitability lies not in incremental improvements, but in a reimagining of how we approach AI implementation and integration.
We present a provocative thesis: the AI community must drastically overhaul its methods to unlock exponential returns. Organizations clinging to traditional AI deployment strategies are leaving vast sums of money on the table. In contrast, those embracing cutting-edge approaches—such as Compound-AI systems, DataIntelligenceOps, Partial Reasoning and LLM-infused MLOps— can see significant ROI increases.
This is not merely about adopting new technologies; it's about fundamentally restructuring how we think about operational AI in business. We argue for a shift from isolated AI projects to enterprise-wide AI diffusion, from rigid maturity models to agile, technology-accelerated implementations. Our findings suggest that companies leveraging real-time, multi-modal data and deep reinforcement learning are not just optimizing processes—they're revolutionizing entire industries.
The stakes are clear: adapt or be left behind. Article suggests a roadmap for visionary leaders ready to transcend the limitations of current AI paradigms and unlock the true, transformative potential of artificial intelligence advances. The $600 billion question isn't just about closing a gap—it's about unleashing a new era of AI-driven profitability.
Section: Introduction:
The emerging disappointments with generative AI ROI are not news. There have been several talks and discussions on this including:
Dave Cahn’s thought-provoking article (https://www.sequoiacap.com/article/ais-600b-question/)
https://www.youtube.com/watch?v=qWf2tWrNSSk.
More subtle questions along the same lines have been raised.
Having said that, it must be pointed out that as AI practitioners, we do not find this surprising – have battled at least half a dozen CFOs about their reticence to invest in AI. The limitations and the progress path of AI are well understood, but my main thesis here is different.
Thesis: The AI community must figure out better and more consistent ways to raise the ROI levels from AI, so that the objections get mitigated, but the resistance to broader AI adoptions are removed. The path to much higher ROI is a set of approaches that have consistently worked across many firms over the last 5-7 years.
Section: 10 point path to Accelerated AI-ROI
1. Start with the Data: Build the next generations of data infrastructure and engineering processes.
o Need for DataIntelligenceOps: Today’s AI apps, especially in Gen-AI, are often geared towards knowledge enablement, reasoning support, decision automation, intelligent search etc. But much of the data infrastructure today is stuck on supporting old style reporting. First, reporting tools themselves are going through a radical shift and the core ingestion, Cloud-EDW dbt like environments will remain important for a while. But we need to think beyond this for the next gen of data engineering.
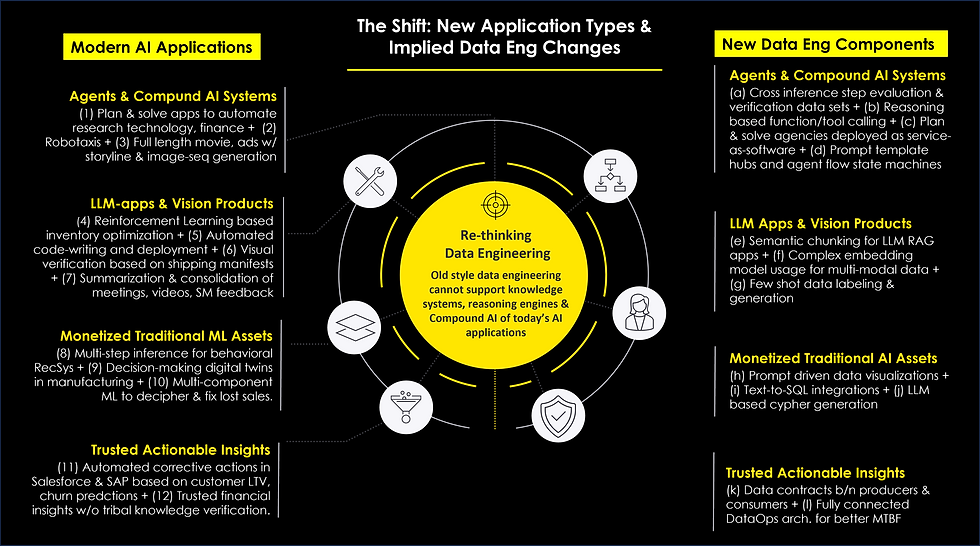
o Impact Example: National auto-parts firm that has stumbled on updating its data infrastructure is unable to use AI for any kind of strategic impact, while its main competitor just introduced a new revenue stream using AI for sustainability.
o Situation: The shift today is driven by the nature of the emerging applications and their characteristics. The graphic here maps the application needs to the consequent artifacts that must appear in our data infrastructure and engineering toolkits.
o Industry Data: [McKinsey] Companies that invest in data infrastructure can see a 20-30% improvement in their data-driven decision-making capabilities. This indicates that a robust data engineering framework is essential for maximizing the potential of AI.
2. End-to-End Industry Systems: Build industry specific end to end systems, including feedback actions, insights into upstream systems (not just downstream systems). Don’t punt after just operationalizing the model.
· Problem: Most firms I have worked with tend to leave the AI work unfinished. Example, they’ll figure out better demurrage options in logistics, but seldom automate the fixing of the process and operations in the transactional systems to actually remove logistics hurdles consistently– essentially, they punt after figuring out the better logistics options. The same thing happens consistently in lost sales recovery, inventory slowdowns, improving shrinking customer LTV.
· Industry Data: Deloitte found that organizations implementing industry-specific AI solutions reported a 30% increase in operational efficiency. Thus, tailored industry systems can provide significant insights and improvements across the entire business process, including upstream systems.
· Fix: The AI approach has to include end to end industry solutions and systems, which include “updates to” and “integration with” transactional systems. Example: integrating supply chains across a conglomerate and an oil super major showed value, only when the SAP processes at both ends were updated with payment optimization insights [professional experience].
3. Spread the net wide: Diffuse the use of AI throughout firms to aggregate ROI from multiple places
· No more Spot AI: The time for piece meal introduction of AI into firms is long past. Economies of scale and internal tribal knowledge necessary for achieving high ROI, just does not come from spot application of AI. Multiple applications, cross-business unit AI projects and data and leverage of learnings, are critical building blocks.
· Industry Data: MIT Sloan Management Review indicates that organizations that integrate AI across various departments (sales, marketing, operations) experience a 40% increase in ROI compared to those that use AI in isolated functions - highlights the value of widespread AI adoption.
· Jamie Dimon Quote: “(JP Morgan) My company has 400 "AI projects" and will continue to grow each year - use cases are in marketing, fraud, and risk. My guess is 800 in a year — 1,200 after that. It is unbelievable for marketing, risk, fraud. Think of everything we do."
· ROI calculator: Most firms, do not actually build ROI calculators for all the AI efforts within their confines. Thus, investment decisions are often left to personal judgements. Usually, a ROI table with (a) specific named projects, (b) investment levels for different modes (c) impact assessment (d) time-lines (e) Risk Costs, is a good beginning.
4. Compound-AI: Compound-AI with multiple inference steps and reach-outs to independent systems is an effective path forward for higher ROI systems
What is Compound-AI: “Shift from models to compound AI systems” (https://bair.berkeley.edu/blog/2024/02/18/compound-ai-systems/) states that "state-of-the-art AI results are increasingly obtained by compound systems with multiple components, not just monolithic models."
Industry Examples: AlphaGeometry: "combines an LLM with a traditional symbolic solver to tackle Olympiad problems". Google's Gemini: Used a "CoT@32 inference strategy that calls the model 32 times" for the MMLU benchmark, achieving 90.04% accuracy compared to 86.4% for GPT-4 with 5-shot prompting.
Industry Example: Pharmaceutical companies are exploring the use of LLMs in combination with other AI models and chemical databases to assist in drug discovery processes, including predicting drug-target interactions and optimizing molecular structures [Insilico Medicine]
Fix: In traditional AI, many of us have used multi-step chains/workflows to get the right results – but this approach applies to Gen-AI systems, too. In the last 7 months, we have used compound Gen-AI steps to solve issues with SC risk optimization and in environment sustainability.
5. Skip a maturity Level: Use technology short cuts to get to shorter paths to revenue.
· Technology Accelerators: Using low code and no-code tools and completely outsourced AI platforms is an efficient way to make the path to AI ROI shorter. For example, there are many of fully managed cloud-based AI-MLOps platforms. Firms can use a “load your data and let the outsourced AI platform firm do the rest” approach to democratize AI. In essence, some maturity steps can be entirely skipped, and others can be accelerated. The resistance to years of internal maturity building for ROI is diminished.
· Industry Data: Gartner indicates that organizations that leverage low-code and no-code platforms can reduce application development time by up to 70%. This can lead to faster revenue generation by accelerating AI maturity buildouts.
· Industry Data: [Managed AI platforms – Domino Data Lab] Customers reported 10x faster model deployment and 70% reduction in infrastructure costs [Source: Domino Data Lab website]
6. Reasoning Systems: Partial reasoning systems are possible, a combination of steps – and they provide tremendous value, even before AGI kicks in.
· Industry Data: Research published in the journal Artificial Intelligence demonstrates that partial reasoning systems can still achieve over 80% accuracy in decision-making tasks. This suggests that even incomplete systems can provide substantial value.
· Example: [Medprompt] This system uses GPT-4 along with nearest-neighbor search and chain-of-thought reasoning to answer medical questions. It outperforms specialized medical models like Med-PaLM when used with similar prompting strategies.
· Example: [Harvey ai] Several law firms and legal tech companies are using LLM-based systems for contract analysis, due diligence, and legal research. These systems often combine LLMs with retrieval from legal databases and domain-specific reasoning.
· Fix: Today, LLM based reasoning systems are possible through a combination of approaches, including (a) Context strengthening (b) Plan & Solve (c) Agentic tool callouts (d) Self-corrective and LLM-judges € Information models such Knowledge Graphs (e) pre-curated prompt templates (f) Enhanced data semantics etc. As can be seen above and in many other industry examples, these approaches when combined together, do start giving us abilities that are fundamentally beyond chatbots, search and content generation.
7. LLM infused MLOps: MLOPs and LLMOps which monitor and self-adjust through corrective actions can increase business values
· Self-Correcting MLOps: A hard problem when production-izing traditional ML and also gen-ai is the need to adjust and remediate to match drifts. Here the reference is not just to data and model drifts, but also business metrics drifts. Usually, most firms do some amount of manual remediation for this, which can be a slow and interrupted process. Self-corrective MLOps and LLMOps and reflective agent flows are excellent tools to ameliorate this issue. Leads to consistently optimized business metrics.
· Industry Example: Microsoft's Project Miyagi uses GPT models to generate entire ML pipelines based on natural language descriptions. Arize AI and Fiddler AI are incorporating generative AI into their MLOps platforms to provide more intelligent insights into model performance and to assist in debugging.
· Examples: [Arize, Octo ML, Data Robot,] Generative AI is being used to enhance anomaly detection in model performance and to provide more detailed root cause analysis when issues arise. Some startups are working on using generative AI to assist in feature engineering, potentially automating parts of the MLOps pipeline.
8. Real World Data: Use real-time and multi-modal data much more aggressively.
· Real World Data Sources: Over the last 8 months, several firms have expressed an interest in using generative AI with multi-modal data including weather data, video news, tabular IoT data etc. Moreover, many of these data sources force us to work with real-time streams. This in itself is not a difficult concept, but it is necessary for the industry to step up to these data sources, thus extending the reach (and hence ROI) of LLM-apps. It’s time to shift focus away from just pdfs.
· Small Fix: Providing appropriate Knowledge Graph Embeddings for multi-modal data opens up new LLM-apps. (See https://www.dakshineshwari.net/post/multi-modal-knowledge-graph-embeddings)
· Industry Data: Accenture found that organizations using real-time analytics and multi-modal data (combining structured and unstructured data) achieve a 30% increase in customer satisfaction and a 20% increase in revenue – thus, underscoring the importance of leveraging diverse data sources effectively.
9. Productivity increases: When done at scale.
· Industry Insights: Carlini in his wonderful article lists various ways, he uses AI (gen-ai): (a) Building entire webapps with technology I've never used before. (b) Teaching me how to use various frameworks having never previously used them. (c) Converting dozens of programs to C or Rust to improve performance (d) Trimming down large codebases to significantly simplify the project. (e) Writing the initial experiment code for nearly every research paper I've written in the last year. (f) Automating nearly every monotonous task or one-off script (g) Almost entirely replaced web searches for helping me set up and configure new packages or projects. (h) About 50% replaced web searches for helping me debug error messages [See https://nicholas.carlini.com/writing/2024/how-i-use-ai.html]
· Industry Data: Salesforce Reported that their Einstein GPT AI assistant increased sales productivity by 28% for early adopters. [ Source: Salesforce, "Einstein GPT: The World's First Generative AI for CRM" (2023)]
· Industry Data: Coca-Cola: Reported that their use of generative AI in marketing campaigns led to a 15-30% increase in advertising effectiveness. - Source: The Drum, "Coca-Cola says generative AI has boosted its ad effectiveness by up to 30%" (2023)
· Fix: Consistently find that even technical people do not know how to increase their productivity radically. But, at scale productivity increases across firms can lead to significant gains, as JP Morgan is seeing. This training and change in internal processes do help. To refine thus further, some firms are adopting prompt template repositories and hubs (see langhub)
10. Deep Reinforcement Learning to the rescue: Use reinforcement learning to significantly improve internal processes – cost savings can be revolutionary.
· Problem: Process inefficiencies can exact a heavy toll on internal expenses. This is especially true for supply chain processes. For example: an end to end procure-to-pay process may take weeks including human intervention, reducing the pace at which a firm operates. But deep reinforcement learning is an excellent approach to solve such problems.
· Industry Data: Alibaba: Used deep reinforcement learning to optimize its supply chain and logistics operations. Reported a 10% reduction in vehicle mileage and a 30% improvement in efficiency of its logistics operations. Source: MIT Technology Review, "Alibaba is using AI to optimize its supply chain" (2018)
· Industry Data: JD.com: - Implemented DRL for inventory management and demand forecasting. - Achieved a 15% reduction in inventory costs and a 20% improvement in forecast accuracy. - Source: Forbes, "How JD.com Uses AI, Big Data & Robotics to Take on Amazon" (2018)
· Industry Data: Unilever: Implemented DRL for demand forecasting and inventory optimization. Achieved a 20% reduction in inventory levels and a 2-3% increase in sales due to improved product availability. [Source: Supply Chain Digital, "Unilever: digital transformation in supply chain" (2020)]
· Approach: Paper by Amazon on “Deep Inventory Management” apart from presenting eye-popping results, also outlines the implementation and experimental setup quite well. (See [2210.03137] Deep Inventory Management (arxiv.org))
Section: What does a high-ROI AI system mean?

The attached case study components have been modified slightly, to hide the identity of the firm. Essentially the figure talks through the business complexities involved and hence the business metrics that were measured, the inference steps and data enhancements refer to the compound-AI steps and technologies used. The platform and tools are a good measure of the overall complexity of the efforts. It also helps decide if the work should be done in an outsourced PaaS model or in-house.
Section: Conclusion:
The focus on improving infrastructure, chips and models will lead to quantum jump in technology progress, but without enabling a more composite path to increased ROI, much of the viability of AI will not be realized. The problem as noted, is not that firms are not investing in generative AI, but often lack the wherewithal to realize the right levels of ROI.
Perhaps the biggest stumbling block amongst both CXOs and CTOs is often a poor understanding of the “Realizable art of the possible” (not just the art of the possible). Thus, if you don’t know that converting financial data into images and using vision transformers can lead to good results, you’ll never reach the potential ROI gains of using such techniques.
Arindam Banerji (banerji.arindam@gmail.com)
Comments